The nice thing about working on a team with other analysts, working as part of a research group, or working on your PhD is the ability to share analysis with other colleagues, get feedback, and learn new ways of thinking about things.
Once the package is installed, using the same Python distro that is used for your Jupyter Notebook/JupyerLab, you can convert a R Markdown file by following these steps: Open a terminal (Unix) or a command line window (Windows). Navigate to your script.Rmd file location. Run jupytext -to notebook script.Rmd to have a script.ipynb file. Turn your analyses into high quality documents, reports, presentations and dashboards with R Markdown. Use a productive notebook interface to weave together narrative text and code to produce elegantly formatted output. Use multiple languages including R, Python, and SQL. R Markdown supports a reproducible workflow for dozens of static and dynamic output formats including HTML, PDF, MS. Data Analysis Template in R Markdown & Jupyter Notebook The nice thing about working on a team with other analysts, working as part of a research group, or working on your PhD is the ability to share analysis with other colleagues, get feedback, and learn new ways of thinking about things.
The Notebook ribbon appears automatically when the Notebook view is open. Refer to the official Jupyter Notebook user interface documentation for details on how to interact with Jupyter Notebooks. Additional help. The Notebook view help menu provides a tour of the Jupyter Notebook user interface, keyboard shortcuts, Markdown, and other relevant.
Interestingly, when I’ve inquired to colleagues at some teams about how they share their analysis with their group they often say that, “people do their analysis and just present the results”. I think this is a big miss in terms of being able to have transparency in the research process, sharing so that others can learn or help to provide constructive feedback, and walking through the steps you went through (data retrieval, data cleaning, analysis, model testing, etc) to ensure that things make sense to the group before being shared with the end user.
For the PhD student, a more streamlined approach to the entire analysis can help them talk through what they did with their advisors, ensure that all the correct steps were taken during the analysis, and have greater confidence about what their data is and is not saying (which can really come in handy when it is time to defend the thesis!). When I was doing my PhD I would often try and put all my steps into a power point presentation to walk through with my supervisors. I never liked that, however, because it always felt clumsy and I was never really getting to the guts of the analysis as much as I was just sharing the outcomes of what I did and why I did it and talking through how I did it. A template that allows for a clear presentation would have made things much easier for both myself and my supervisors
In my last post, I used R Markdown to create a report that allows the sport scientist to share some basic data analysis with the strength and conditioning staff and other coaches. As I said in that post, R Markdown is a wonderful resource for creating reports where you can hide your code and simply show visualizations of the data and model outputs. But, what if we don’t want to hide our code?! In this sense, R Markdown is extremely useful for setting up a data analysis template to allow you to walk through all the steps in your project and share the results with your colleagues or PhD supervisors. Additionally, you could also keep R Studio open when presenting your findings and address any changes/suggestions that people may have, in real time before, “knitting” the markdown file into the final html or pdf document. This last part allows the analysis to come to life and allows you to make direct changes and immediately show how they impact the outcome of the analysis!
Data Analysis Templates
There are a number of different data analysis frameworks one could follow. Is half life 2 for mac. Two that immediately come to mind are the Cross Industry Standard Process for Data Mining (CRISP-DM) and the Problem, Plan, Data, Analysis, and Conclusion (PPDAC) Cycle.
Although they come from different industries — CRSIP-DM from the business world and PPDAC from more of the statistics education world — there is considerable overlap and both have the aim of providing the analyst with a clear path to answering their research question.
The objectives of each phase within these two frameworks is shown below.
As you can see, the end goal of the analysis is different between the two frameworks: CRISP-DM being targeted at deploying a model specific to business use cases and PPDAC providing more of a runway for scientific publication. However, both can provide us with an appreciation for creating a systematic process around data analysis, allowing for a clear explanation of our approach when discussing with colleagues or PhD supervisors.
In an attempt to create something more generic and less specific to a certain industry or field, I came up with my own framework:
The framework is freely available on my GitHub page in both an R Markdown and Jupyter Notebook (if you prefer Python) formats. If you’d like to see with the R Markdown HTML looks like, click here >> PWard_-_Data_Analysis_Framework.
All you have to do is take the template (either R Markdown or Jupyter Notebook), delete the comments that I have under each section and fill in your own comments and your R or Python script, where applicable, to conduct your analysis. Once complete, you will have a clean looking file that details your entire approach.
I’ve made a simple example of what using the template could look like. If you are interested in seeing the result in R Markdown, CLICK HERE >> Data_Analysis_Framework_Example_–_MLB_Hitting. If you are interested in seeing the result in a Python Jupyter Notebook, CLICK HERE >> Data Analysis Framework Example — MLB Hitting (Jupyter).
All of the code and the templates for use are available on my GitHub page.
Markdown
format is.Markdown
for documentation of earth data science workflows.Markdown
syntax in Jupyter Notebook
to:Markdown
is a human readable syntax (also referred to as a markup language) for formatting text documents. Markdown
can be used to produce nicely formatted documents including PDFs and web pages.
When you format text using Markdown
in a document, it is similar to using the format tools (e.g. bold, heading 1, heading 2) in a word processing tool like Microsoft Word or Google Docs. However, instead of using buttons to apply formatting, you use syntax such as **this syntax bolds text in markdown**
or # Here is a heading
.
Markdown
syntax allows you to format text in many ways, such as making headings, bolding and italicizing words, creating bulleted lists, adding links, formatting mathematical symbols and making tables. These options allow you to format text in visually appealing and organized ways to present your ideas.
You can use Markdown to format text in many different tools including GitHub.com, R using RMarkdown, and Jupyter Notebook, which you will learn more about this page.
Data Tip: Learn more about how you can use Markdown to format text and document workflows in a variety of tools.
A great benefit of Jupyter Notebook
is that it allows you to combine both code (e.g. Python
) and Markdown
in one document, so that you can easily document your workflows.
A Jupyter Notebook
file uses cells to organize content, and it can contain both cells that render text written using the Markdown
syntax as well as cells that contain and run Python
code.
Thus, you can use a combination of Markdown
and Python
code cells to organize and document your Jupyter Notebook
for others to easily read and follow your workflow.
Data Tip: Learn more about Markdown for Jupyter Notebook.
If you render your Jupyter Notebook
file to HTML or PDF, this Markdown
will appear as formatted text in the output document.
Data Tip: In fact, this web page that you are reading right now is generated from a Markdown
document! On this page, you will learn the basic syntax of Markdown
.
Being able to include both Markdown
and code (e.g. Python
) cells in a Jupyter Notebook
file supports reproducible science by allowing you to:
all in one document!
When used effectively, Markdown
documentation can help anyone who opens your Jupyter Notebook
to follow, understand and even reproduce your workflow.
In the previous chapter on Jupyter Notebook
, you learned how to add new Markdown
cells to your Jupyter Notebook
files using Menu tools and Keyboard Shortcuts to create new cells.
Function | Keyboard Shortcut | Menu Tools |
---|---|---|
Create new cell | Esc + a (above), Esc + b (below) | Insert→ Insert Cell Above OR Insert → Insert Cell Below |
Copy Cell | c | Copy Key |
Paste Cell | v | Paste Key |
You also learned how to change the default type of the cell by clicking in the cell and selecting a new cell type (e.g. Markdown
) in the cell type menu in the toolbar. Furthermore, you learned that in a Jupyter Notebook
file, you can double-click in any Markdown
cell to see the syntax, and then run the cell again to see the Markdown
formatting.
Note: if you type text in a Markdown
cell with no additional syntax, the text will appear as regular paragraph text. You can add additional syntax to that text to format it in different ways.
On this page, you will learn basic Markdown
syntax that you can use to format text in Jupyter Notebook
files.
You can create a heading using the pound (#
) sign. For the headers to render properly, there must be a space between the #
and the header text.
Heading one is denoted using one #
sign, heading two is denoted using two ##
signs, etc, as follows:
Here is a sample of the rendered Markdown
:
Note: the titles on this page are actually formatted using Markdown
(e.g. the words Section Headers above are formatted as a heading two).
You can also use Markdown
to create lists using the following syntax:
It will render as follows:
Notice that you have space between the *
or 1.
and the text. The space triggers the action to create the list using Markdown
.
You can also use **
to bold or *
to italicize words. To bold and italicize words, the symbols have to be touching the word and have to be repeated before and after the word using the following syntax:
It will render as follows:
These are italicized words, not a bullet listThese are bold words, not a bullet list
If you want to highlight a function or some code within a plain text paragraph, you can use one backtick on each side of the text like this:
which renders like this:
Mdma for mac os. Here is some code!
The symbol used is the backtick, or grave; not an apostrophe (on most US keyboards, it is on the same key as the tilde (~)).
You can also create a horizontal line or rule to highlight a block of Markdown
syntax (similar to the highlighting a block of code using the backticks):
which renders like this:
Here is some important text!
You can also use HTML in Markdown
cells to create hyperlinks to websites using the following syntax:
<a href='url' target='_blank'>hyperlinked words</a>
You can identify the words that will be hyperlinked (i.e. prompt a web page to open when clicked) by replacing hyperlinked words
in the example above.
For example, the following syntax:
Our program website can be found at <a href='http://earthdatascience.org' target='_blank'>this link</a>.
will render as follows with this link
as the hyperlinked words:
Our program website can be found at this link.
You can also use Markdown
to link to images on the web using the following syntax:

The alt text is the alternative text that appears if an image fails to load on webpage; it is also used by screen-reading tools to identify the image to users of the screen-reading tools.
For example, the following syntax:
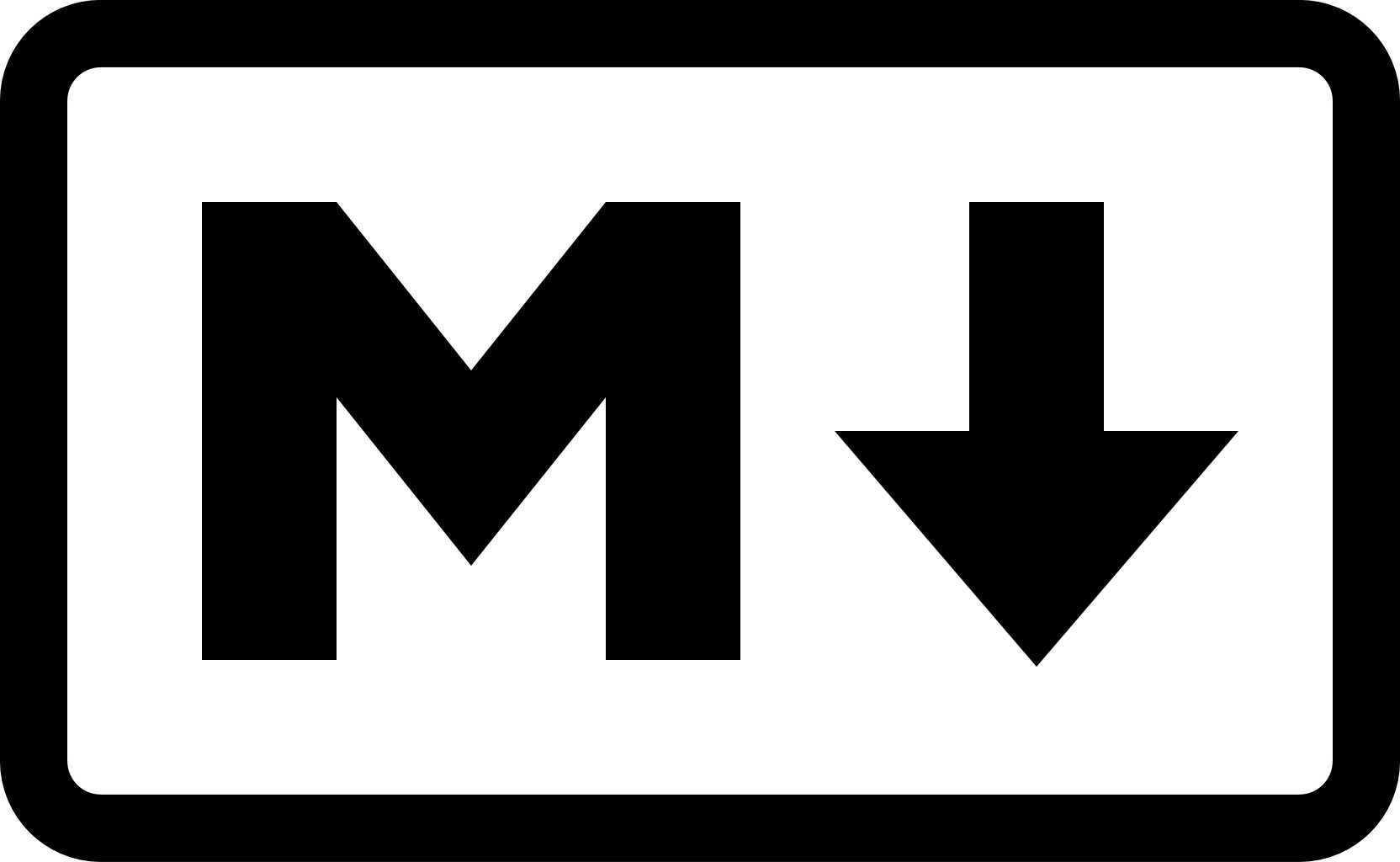
will render as follows with an alt text of Markdown Logo is here.
:
You can also add images to a Markdown
cell using relative paths to files in your directory structure using:

For relative paths (images stored on your computer) to work in Jupyter Notebook
, you need to place the image in a location on your computer that is RELATIVE to your .ipynb
file. This is where good file management becomes extremely important.
For a simple example of using relative paths, imagine that you have a subdirectory named images
in your earth-analytics
directory (i.e. earth-analytics/images/
).
If your Jupyter Notebook
file (.ipynb
) is located in root of this directory (i.e. earth-analytics/notebook.ipynb
), and all images that you want to include in your report are located in the images
subdirectory (i.e. earth-analytics/images/
), then the path that you would use for each image is:
images/image-name.png
If all of your images are in the images
subdirectory, then you will be able to easily find them. This also follows good file management practices because all of the images that you use in your report are contained within your project directory.
Data tip: There are many free Markdown
editors out there! The atom.io editor is a powerful text editor package by GitHub, that also has a Markdown
renderer that allows you to preview the rendered Markdown
as you write.
Open or create a new Jupyter Notebook
file.
Markdown
cell and include:Intro to Earth Analytics - Chapter Four
)Author:
and then add text for your name.Date:
and then add text for today’s date.Markdown
cell and include: